Custom Clinical Decision Support System (CDSS)
Architecture, Development Steps, & Costs
In custom healthcare software development since 2005, ScienceSoft builds robust, regulatory-compliant systems to help clinicians make informed decisions on diagnosing, treatment, admission, and other aspects of patient care.
Clinical decision support system (CDSS) is needed to suggest possible diagnoses, optimal treatment options, and administrative actions, help validate the appropriateness of medical procedures, and provide clinicians with access to clinical and evidence-based guidelines. Such systems can also help identify medication interactions, at-risk patients, and patient non-compliance with treatment plans.
Custom clinical decision support software is an optimal solution for healthcare organizations with complex clinical workflows and IT landscapes. Custom solutions can be integrated with multiple existing systems across different departments and tailored to assist in diagnosing, monitoring, and treating specific conditions. They are also suitable for organizations that want to adopt ML/AI-powered features, such as medical image analysis or NLP/LLM-powered data search.
- Implementation time: 6 to 18+ months.
- Common integrations: EHR/EMR, LIMS/LIS, PACS, RIS, remote patient monitoring software, databases of organizations that publish medical research.
- Costs: $300,000 to $3,000,000+, depending on the solution's complexity. Use our free online calculator and get a custom quote from our consultants.
Clinical Decision Support Market Size Is To Reach $4.46 Billion by 2030
According to Mordor Intelligence, the major driver of increasing CDSS adoption is the strong focus of the healthcare industry on value-based care, prioritizing quality and positive health outcomes. The tendency calls for advanced, reliable decision-making tools.
High-Demand CDSS Features
CDSS can compare patient symptoms against information in a clinical knowledge base (e.g., clinical guidelines, best practices) and a patient’s medical history and then create a list of potential diagnoses. The system can also be powered by AI/ML algorithms, e.g., to predict the risk of specific conditions, interpret lab test results, analyze medical images such as CT scans, MRIs, X-rays.
Order entry support
A clinical decision support solution can assist with accurate test and procedure ordering relevant to a specific patient's diagnosis. The system can recommend predefined condition-specific order sets, evaluate the appropriateness of imaging orders (e.g., in line with Appropriate Use Criteria [AUC]), and provide information on the cost implications of different choices.
Medication selection support
A CDS system can generate alerts on possible allergic reactions to the prescribed medication, check drug interactions and adverse events, identify cases of duplicate therapy, and determine the optimal dosage based on the patient's individual characteristics and comorbidities. The software can also suggest an alternative medication option if it detects prescription discrepancies.
Condition-specific recommendations
The system can generate care plans (e.g., for oncology, chronic diseases) with proven treatment options, patient-specific recommendations (e.g., based on medical history, demographics, genomic profile). The clinician can then evaluate the options and choose the most appropriate one.
Patient monitoring
A CDSS can feature capabilities for onsite and remote monitoring of patient vital signs (e.g., blood glucose level, blood pressure) and sending alerts when a patient's condition deteriorates. The deviation thresholds and periods for the alerts can be customized to align with clinical protocols and minimize unnecessary alerts. The system can also provide historical analytics on vital parameters to identify trends and evaluate the effects of medical interventions.
Admission decision support
A CDSS can analyze patient data (e.g., vital signs, symptoms, lab test results), condition-specific clinical guidelines, and bed and staff availability to help healthcare professionals make evidence-based admission decisions.
Emergency department triage support
CDSS can compare patient health data (e.g., vitals and symptoms) against defined criteria, determine the urgency of care needed, and automatically flag priorities. Integrated with other hospital systems (e.g., hospital asset tracking system), CDSS can also factor in real-time resource availability, like operating room status.
Clinical management support
CDSS may provide care management recommendations according to the latest clinical guidelines and research. For example, it can suggest protocol-specific procedures, preventive care actions (e.g., vaccination and screenings), and timely follow-up appointments. The system can also send alerts on deviations from clinical guidelines within the treatment plans, patient non-compliance with the prescribed care, and delayed diagnostic tests.
Collaboration and information management tools
CDSSs can be accessed directly via the EHR/EMR and supplied with complementary tools, such as collaborative workspaces for creating treatment plans and managing care and AI-powered chatbots for summarizing clinical notes.
Clinical trial matching support
A CDSS can analyze patient medical history data, including specific genetic markers, and suggest relevant clinical trials to help patients access alternative treatment options while streamlining clinical trial recruitment for CROs and other research organizations.
An analytics component can help identify inefficiencies in care delivery (e.g., prolonged hospital stays due to delayed interventions, duplicate testing). It can also predict patient deterioration risks based on historical data and real-time vitals and compare the effectiveness of treatment options for specific conditions (e.g., sepsis management strategies). Additionally, it can generate reports for C-level executives on clinical performance metrics and for regulatory bodies on compliance with treatment guidelines and quality standards.
Radiation dosage monitoring
The software can analyze the administered radiation dosages and gauge their safety depending on patient-specific parameters (e.g., previous exposure, age). Based on the analysis, the system will flag an inappropriate dosage and recommend a safe one.
See How Our Clients Benefit From Custom CDSS Solutions
8 results for:

CE-Marked Laboratory Diagnostics Software Delivered in 7 Months
ScienceSoft designed and developed laboratory diagnostics software for quality assurance and interpretation of genetic screening results.

New Features for Medical Imaging SaMD Driving 3x User Growth
ScienceSoft delivered a compliant web demo of radiographic image analysis software for a global provider of AI-based medical imaging solutions. The demo showcases the SaMD’s capabilities and helps attract potential clients by enabling the product’s free trial.

Lung Cancer Detection Application for bioAffinity Technologies
In 2 months, ScienceSoft provided a US-based biotechnology company with a desktop app for lung cancer detection. The app ensures 100% stable generation of comprehensive diagnostic reports based on analysis of flow cytometry data.

MRI Scans Analysis to Detect Brain Tumors Using CNN Algorithms
ScienceSoft created a CNN-based application to automatically analyze brain MRI scans, localize tumors, and define each tissue type.

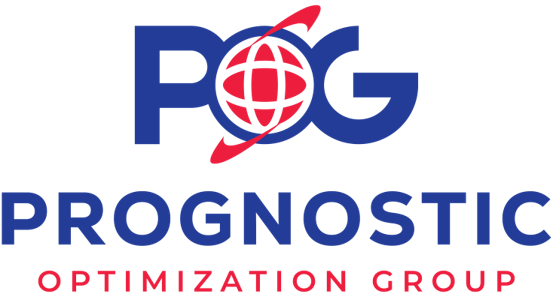
AI Telehealth Chatbot PoC and Investor Pitch Deck for Prognostic Optimization Group
In two weeks, ScienceSoft delivered a PoC for a US startup’s AI telemedicine chatbot meant to streamline patient diagnostics and provide clinical decision support to physicians. The startup also received clickable UI prototypes and an engaging pitch deck for investors.
Sample Architecture of a Clinical Decision Support Solution
Below, our software architects provide a sample architecture a clinical decision support system.
A clinical decision support system generates output (e.g., recommendations, alerts) based on data from the integrated systems (e.g., EHR/EMR, remote patient monitoring software, clinical knowledge base of best practices and guidelines). The system can also rely on data from external data sources such as medical research databases (e.g., PubMed, Cochrane Library, UpToDate), pharmacology databases (e.g., RxNorm, Micromedex, FDA Orange Book), and AI-powered research aggregators (e.g., Semantic Scholar, LitCovid). Integration with CPOE (computerized provider order entry) helps clinicians electronically enter medication, laboratory, and radiology orders.
Depending on the use case, different systems can be integrated with a CDSS directly or via EHR. Direct integration is optimal when real-time output is needed, while EHR-based integration is best for historical analysis. However, both options have their downsides. Too many direct integrations require additional format standardization efforts, but when all systems are connected through EHR software, the latter affects CDSS performance. In most scenarios, a hybrid approach can help strike a balance. For example, we can directly integrate CDSS with cardio monitors to receive real-time alerts on abnormal heart rates and rely on the integration with EHR to access historical heart state data needed for generating personalized treatment recommendations.
The inference service is responsible for processing knowledge artifacts from the databases and applying them to case-specific patient data. For example, a patient is prescribed a certain medication, and the recent lab test result indicates this patient has renal function impairment, which could increase the likelihood of an adverse drug reaction. The role of the inference service is to compare the prescribed drug against the list of interactions and related medical conditions and then issue an alert if the medication is deemed inappropriate. The service can be powered by rule-based mechanisms and an ML/AI-driven engine. The ML/AI block can also be responsible for other intelligent tasks, such as medical image analysis and data search based on natural language queries.
A data warehouse stores highly structured data that is used by the analytics service to generate reports and forecasts. The ML/AI engine processes the DWH data to find patterns and get new data for self-learning.
Several services within the system ensure its security and compliance with data protection regulations like HIPAA, GDPR, PDPL, and PIPEDA. The user management service handles user profiles, roles, and permission and features user access and authentication mechanisms. The data protection service ensures data encryption at rest and in transit, data anonymization and de-identification, and other mechanisms that prevent sensitive data loss and exposure. The audit and logging service keeps records of user actions, changes to patient data, and system events to ensure complete transparency and accountability of actions within the system.
How To Develop Custom CDSS: Expert Tips and Best Practices
Custom CDSS development is needed when an organization wants to integrate the system into highly specific clinical workflows, achieve smooth system interoperability with other back-office software, and combine a unique set of features. In custom healthcare software development since 2005, ScienceSoft outlines the key steps for successfully implementing a custom CDSS.
1.
Business analysis
If the CDSS is intended for internal use, business analysts collaborate with the stakeholders (e.g., physicians, nurses, administrative staff, CIO) to elicit the details essential for shaping functional and non-functional solution requirements. The experts clarify what types of decisions are to be supported by the software (e.g., diagnosing, order entry) and what systems and workflows the organization has in place.
If the software is to be distributed commercially, business analysts typically interview the product owners, understand their vision of the product, identify the needs of the target organizations, perform competitive market analysis, and decide on solution features that will help win the competition.
At this stage, compliance consultants identify the applicable compliance regulations that must be met (e.g., HIPAA, GDPR, PDPL for personal data protection).
According to FDA regulations, some CDSSs can be classified as Software as a Medical Device (SaMD). This can be the case when the system provides risk scores, gauges the probability of a disease or condition, or analyzes medical images or continuous signals. Such software must undergo FDA clearance and comply with IEC 62304:2006/Amd 1:2015, ISO 1497, and ISO 13485 standards. Additionally, if the system is to confirm the necessity of an advanced diagnostic imaging service (e.g., CT, MRI, PET) for Medicare patients, it should be built in line with a range of AUC (Appropriate Use Criteria) program requirements. For instance, it must generate compliance certificates for claims submitted to CMS (Centers for Medicare and Medicaid Services) and have ISO 62366-compliant UI.
2.
Technical design
During this stage, solution architects plan architecture components to power the defined workflows and choose techs that satisfy scalability, elasticity, performance, and other requirements at the best cost-to-benefit ratio. They also draw an integration plan and determine standards for enabling data exchange between CDSS and existing hospital systems (e.g., HL7, FHIR).
If a CDSS relies on a knowledge base consisting of clinical guidelines, evidence-based rules, and research to provide decision support, it is essential to make sure that this data is smoothly integrated into the system and is updated based on new findings. This can be done by encoding clinical guidelines according to standardized formats such as the Clinical Decision Support Hooks or the Arden Syntax, creating scenario-specific medical ontologies (e.g., SNOMED CT, LOINC) for easier guidelines mapping, establishing partnerships with organizations that publish evidence-based materials to ensure dynamic data updates.
3.
UI/UX design
UI and UX designers create visual elements and workflows to facilitate smooth navigation and intuitive user experience for physicians. For example, UI designers can use a uniform color scheme across different data types (e.g., blue as normal and red as critical both for alerts and for patient vitals).
One of the major problems in the UI/UX design for clinical decision support software is alert fatigue. When there are too many notifications from the CDSS, and they mix up with messages from other systems, healthcare professionals are likely to ignore some of the alerts that might be crucial for providing quality patient care. To mitigate this problem, UX designers can create concise messages with action recommendations like “Consider changing the medication” or “See patient medical history update”. They can also suggest composing summaries of non-critical alerts and sending them in batches at the end of the day instead of distracting clinicians’ attention with every new message. It should be noted that UX designers are not the only experts responsible for minimizing alert fatigue — other roles can also contribute to it. For example, business analysts can collaborate with clinical stakeholders to classify alerts based on severity, while developers can set group-specific parameters for triggering alerts.
5.
Development and testing
Developers typically try to find ways to optimize development costs while maintaining high software quality. They can reuse ready-made components and services of reputable cloud providers (e.g., Azure, Amazon, Google Cloud), use feasible QA automation, and implement DevOps practices. In ScienceSoft’s projects, this approach has helped reduce development costs by up to 78%.
In many cases, testing is conducted in parallel with development, which helps spot and fix issues early on and thus minimize defects in production.
5.
Deployment and support
The development team deploys the solution and monitors its performance. For a knowledge-based CDSS, they may need to ensure that clinical rules and guidelines remain up to date and that inference logic produces accurate recommendations. For an AI/ML-driven CDSS, they need to evaluate the model’s performance and provide additional training if needed. Developers provide users with software guides and create documentation such as API usage instructions and software maintenance guides. If CDSS is classified as SaMD, the documentation should include the one relevant for submission to regulatory bodies.
How Much Does It Cost To Develop a Custom CDSS?
The cost of custom CDSS development may range from $300,000 to $3,000,000+. The major cost factors include the number and complexity of software features and integrations, the variety of user roles, the need for ML/AI-powered and big data techs, whether CDSS will be considered SaMD or not, and more.
|
Basic solution |
Medium-complexity solution |
Advanced solution |
---|---|---|---|
The number of features
?
E.g., diagnostic support, triage support. |
1 |
2 – 3 |
4+ |
The complexity of decision algorithms
|
Knowledge-based algorithms, where recommendations are powered by matching patient data with user-defined rules and guidelines. |
|
Algorithms of the previous tiers. |
The complexity of integrations
|
Integrates with EHR/EMR for access to comprehensive patient information. |
|
|
Classified as Software as a Medical Device (SaMD)
?
If the system is classified as SaMD, additional efforts may be required to prepare documentation for regulatory submission (e.g., to the FDA). |
|
|
|
The number of users
|
Up to 1,000 |
Up to 5,000 |
5,000+ |
The number of user roles
?
Different user roles require dedicated software workflows and tools. |
1 – 2 E.g., physicians and specialty professionals such as radiologists and pathologists. |
3 – 5 E.g., healthcare professionals and clinical administrators such as clinical managers and quality assurance administrators. |
5+ E.g., healthcare professionals, clinical administrators, data analysts, clinical researchers, and public health researchers. |
Analytics and reporting
|
KPI monitoring (e.g., recovery rates, time to decision) and segmentation (e.g., supported decisions by type). |
|
|
ML/AI-powered data search capabilities
|
|
NLP-powered data search across knowledge bases to retrieve data entries and files based on natural language commands. |
LLM-powered data search across knowledge bases to get summarized information based on natural language commands. |
Software costs
|
$300,000-$600,000 |
$600,000-$1,000,000 |
$1,000,000-3,000,000+ |
Why Build Your CDSS With ScienceSoft?
- Since 2005 in healthcare IT with 150+ successful projects in the domain.
- Experience in achieving compliance with regulations on data protection (e.g., HIPAA, GDPR, PDPL, PIPEDA), ML/AI-powered systems (e.g., GMLP, ISO/IEC 23894:2023, and ISO/IEC 42001:2023), and SaMD (e.g., FDA, MDR, ISO 13485, IEC 62304, ISO 14971).
- Since 1989 in AI and machine learning
- Since 2013 in image analysis software development with the expertise acknowledged in 2022 market leader research by MarketsandMarkets and Coherent Market Insights.
- Proficiency in healthcare data exchange standards, including HL7, DICOM, ASTM, and more.
Our Awards and Recognitions
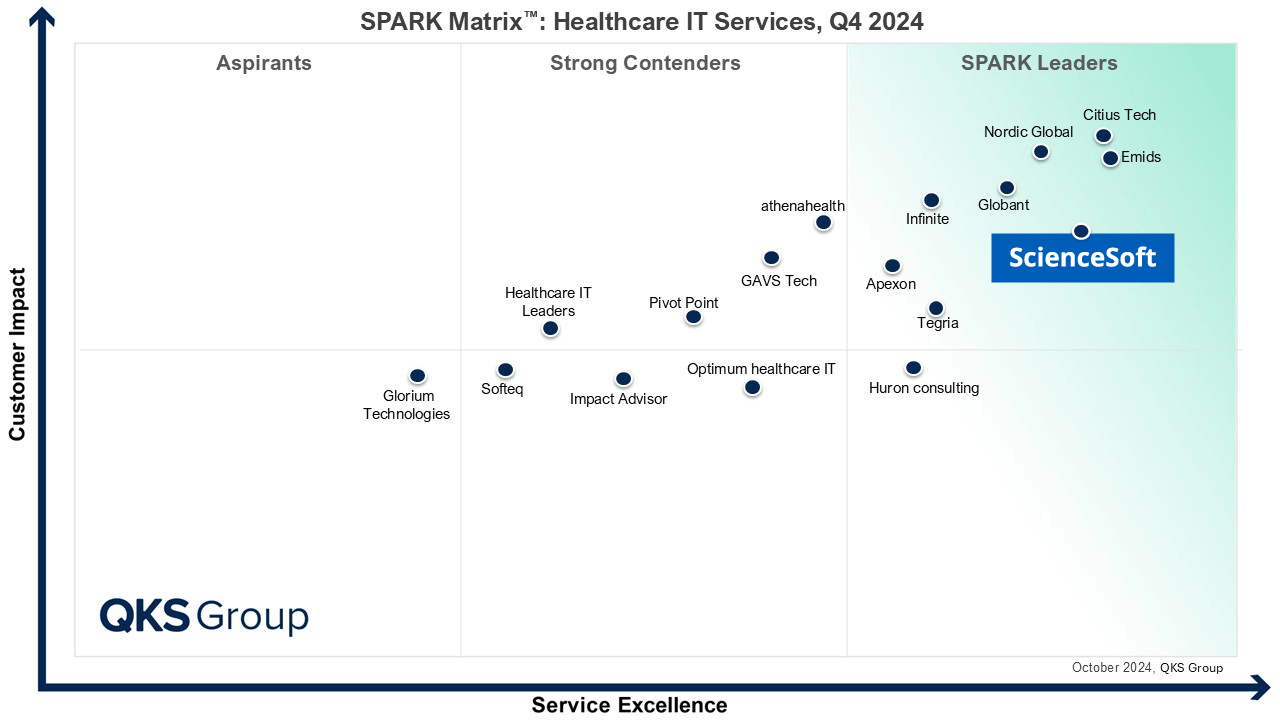
Listed among Healthcare IT Service Leaders in 2022 and 2024
Growing faster than Amazon, Google, and ServiceNow
Recognized for reliability and trustworthiness
Recognized by Health Tech Newspaper awards for the third time

Top Healthcare IT Developer and Advisor by Black Book™ survey 2023
Best in class in medical device connectivity (2023)
A top outsourcing provider for three consecutive years
ISO 13485-certified quality management system
ISO 27001-certified security management system
What makes ScienceSoft different
Driving success in healthcare IT projects no matter what
ScienceSoft develops healthcare IT solutions that reduce care delivery costs and improve outcomes, no matter the challenges posed by diverse expectations of medical staff, shifting priorities, and resistance to change.